Uncovering the Realities of Google's Gemini: Are the AI Models Overhyped?
Jun-30-2024
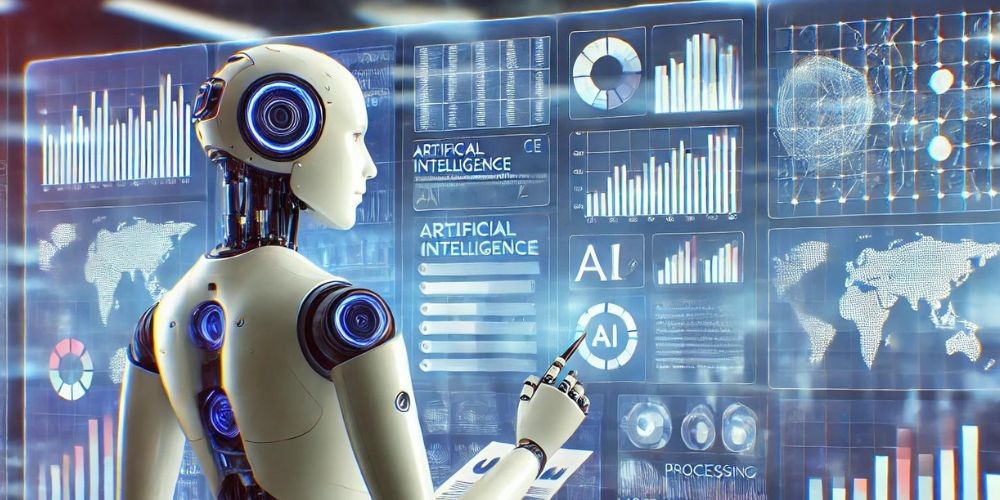
Google has praised its latest AI products, Gemini 1.5 Pro and 1.5 Flash, as revolutionary in their ability to process and analyze massive amounts of data. Promising capabilities such as summarizing hundred-page documents and searching across scenes in videos have attracted attention. However, recent research challenges these claims, revealing that these models might stumble when tasked with making sense of lengthy datasets. A closer examination exposes potential gaps between Google's marketing and the actual capabilities of Gemini's models.
Two studies set out to put Google's assertions to the test. One study, spearheaded by researchers from UMass Amherst, the Allen Institute for AI, and Princeton, focused on how well Gemini's models answered true/false questions about modern fiction books. These were chosen to ensure no prior knowledge could influence outcomes. Unfortunately, results showed Gemini 1.5 Pro and Flash struggled, scoring correct answers just 46.7% and 20% of the time, respectively. Such results suggest significant room for improvement in understanding extensive text passages.
Another study by researchers at UC Santa Barbara evaluated the Gemini 1.5 Flash's prowess with video content. This study tasked the AI with analyzing images within slideshows to answer specific questions. Here, the model's performance was also underwhelming, achieving accuracy rates of just 50% when transcribing six handwritten digits, which dropped further to around 30% with more complex tasks. These findings cast doubt on Gemini's touted abilities to efficiently search for and understand multimedia content.
Despite these setbacks, the benchmarks used in tests did not incorporate the latest 2-million-token context capabilities of Gemini’s models, examining only the 1-million-token versions. This distinction does not absolve Google’s marketing, however. The company heavily promoted long-context capabilities, claiming practical reasoning and understanding over extensive datasets and media. Critics argue that Google's promotional materials exaggerate the AI’s current abilities, leading to potentially misguided expectations among users and developers.
The portrayal of Gemini models as advanced AI capable of sophisticated data analysis remains contentious. Although statistical claims of processing power might hold, real-world applications show that the models have significant limitations. As the AI industry evolves, it's clear there’s a need for improved benchmarks and transparency to provide realistic expectations. Google’s Gemini AI models, despite their advertised strengths, emphasize the broader challenge in generative AI: balancing innovation hype with actual technological capabilities. Moving forward, both researchers and companies must focus on honest evaluations to ensure these tools truly meet user needs.
Without more rigorous, third-party evaluations and honest advertising, consumer trust in generative AI like Gemini stands on precarious ground. The growing scrutiny from executives and investors alike highlights the urgency of demonstrating concrete, reliable advancements rather than relying on potentialities. Consequently, future AI advancements should prioritize verifiable claims over ambitious yet unattainable, promises to sustain progress in this fast-paced industry.